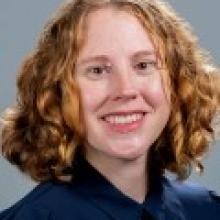
Tracy Becker is an associate professor and the Ed & Diane Wilson Presidential Chair in Structural Engineering at University of California, Berkeley. She has expertise in performance- based design and the design, modeling, and experimental testing of high-performance structural systems used for limiting structural and component losses in seismic events. Her research has focused on expanding the use of seismic isolation to a broader category of structures, understanding ultimate failure mechanisms of isolated buildings to ensure robust designs, and improving existing control systems to further minimize structural responses in seismic events. She received her BS in Structural Engineering from UC San Diego followed by her MS and PhD in Structural Engineering, Mechanics and Materials from UC Berkeley. Afterwards she spent almost two years as a Japanese Society for the Promotion of Science post-doctoral researcher at the Disaster Prevention Research Institute at Kyoto University. Before joining UC Berkeley in 2019, she was an assistant professor at McMaster University in Canada.
Base isolation systems are often chosen in structural engineering to outperform conventional structural designs, allowing designers and owners to achieve high performance goals. However, achieving specific performance targets requires numerous high-fidelity analyses and often involves iteration in design, and analyzing their behavior in extreme events remains a difficult and computationally expensive task, especially when exploring diverse design options. This makes the selection of isolation and superstructure design parameters to achieve targeted performance targets, such as repair cost, downtime, and collapse risk, a time consuming
process. To solve the inverse problem of finding an acceptable design that achieves defined resiliency metrics, a data-driven solution is developed using logistic classification and Gaussian process models. A database of randomly generated isolated building designs under varying ground motion inputs is generated. From the results of the nonlinear analysis, loss and downtime estimation is carried out using the probabilistic methodology prescribed in FEMA P-58. The database is built with design parameters generalized so that the design points are independent of the local seismicity. A logistic classification model is used to determine the probability of the structure experiencing impact against the moat wall, which is particularly important in collapse prediction, and Gaussian process fits are applied to the repair cost and time for the structure. In conjunction, the models return the expected value for the performance target outcomes given the structural design parameters normalized to their demand conditions. The results is the evaluation of the expected loss, downtime, and/or collapse risk over a wide space of generalized designs. This enables the inverse design problem to be solved by limiting the design space to acceptable thresholds. Additional goals, for example minimizing upfront construction cost, can then be used for selecting optimal designs.